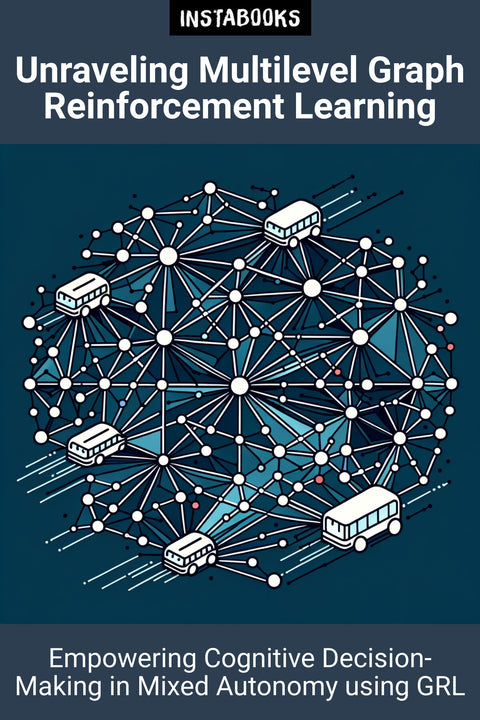
Instabooks AI (AI Author)
Unraveling Multilevel Graph Reinforcement Learning
Empowering Cognitive Decision-Making in Mixed Autonomy using GRL
Premium AI Book (PDF/ePub) - 200+ pages
Introduction to Multilevel Graph Reinforcement Learning
Multilevel Graph Reinforcement Learning (GRL) stands at the forefront of technological innovation, blending hierarchical reinforcement learning with advanced graph structures. This book dives deep into this cutting-edge approach, particularly focusing on its pivotal role in consistent cognitive decision-making within heterogeneous mixed autonomy, such as connected and autonomous vehicles. Explore how GRL transforms complex environments by modeling spatiotemporal interactions and facilitating decision-making with human-like cognition.
Hierarchical Structures and Cognitive Decisions
The core of GRL lies in its hierarchical framework, where it emulates human cognitive functions. This book offers a detailed exposition of how GRL frameworks translate high-level strategies into actionable decisions across various autonomy levels. Readers will uncover how this integration addresses challenges in dynamic environments, ensuring seamless interactions in mixed autonomy traffic scenarios.
Spatiotemporal Dynamics in GRL
Delve into the intricacies of GRL’s ability to model spatiotemporal dynamics, a critical component in environments characterized by unpredictability and high density. The book elucidates upon weighted graph representations that capture these interactions, demonstrating their application in real-world scenarios like highways, where split-second decisions can mean the difference between chaos and order.
Application in Connected and Autonomous Vehicles
Connected Autonomous Vehicles (CAVs) are at the forefront of innovation, benefiting immensely from GRL-based decision-making technologies. This book discusses a generic GRL framework for CAVs, exploring Markov Decision Processes (MDPs) and Partially Observable MDPs (POMDPs) to enable these vehicles to operate with a high degree of autonomy and safety in mixed traffic conditions.
Future of Decision-Making Technologies
Navigate through the future pathways of GRL and its potential impact on autonomous systems. The book covers emerging validation methods, evaluation metrics, and simulation tools vital for advancing GRL technologies. It highlights the ongoing research aimed at overcoming current challenges, enhancing the robustness, scalability, and efficiency of these systems, and opening new avenues for innovation within autonomous technologies.
Table of Contents
1. Introduction to Multilevel Graph Reinforcement Learning- Foundations of GRL
- Recognizing Multilevel Dynamics
- Scope and Potential
2. Hierarchical Structures in Decision-Making
- Cognitive Hierarchies
- Strategic Frameworks
- Actionable Pathways
3. Graph Representations and Interactions
- Modeling Spatiotemporal Interactions
- Handling Dynamic Graphs
- Graph-Based Decision Strategies
4. Cognitive Decision-Making in Mixed Autonomy
- Defining Mixed Autonomy
- Decision Algorithms for Mixed Systems
- Real-World Applications
5. Applications in Connected and Autonomous Vehicles
- GRL Frameworks for CAVs
- Implementing MDPs and POMDPs
- Case Studies and Examples
6. Human-Machine Interactions and Cognition
- Asynchronous Learning Models
- Multi-Head Attention Mechanisms
- Cognition and Adaptability
7. Validation in GRL Systems
- Current Evaluation Methods
- Simulations and Tools
- Challenges and Solutions
8. Future Research Directions
- Emerging Trends in GRL
- Scalability and Efficiency Challenges
- Uncharted Territories in GRL
9. Navigating Complex Environments with GRL
- Dynamic Spatial Correlations
- Temporal Interaction Management
- Innovative Approaches to Challenges
10. Integrating Human-Like Cognition in Autonomous Systems
- Mimicking Human Decision-Making
- Cognitive Models in Action
- Evaluating Cognitive Depth
11. Impact of GRL on Autonomous Technologies
- Long-Term Implications
- Technological Evolution
- Case Studies
12. Concluding Insights and Future Potential
- Summarizing Key Learnings
- Insights from Experts
- Path Forward in Research and Development
Target Audience
This book is aimed at researchers, engineers, and students in the fields of machine learning and autonomous systems, as well as professionals working on decision-making technologies in autonomous vehicles.
Key Takeaways
- Comprehensive understanding of Multilevel Graph Reinforcement Learning and its applications.
- Insights into hierarchical reinforcement learning and cognitive decision-making frameworks.
- Strategies for implementing GRL in connected and autonomous vehicles.
- Exploration of spatiotemporal modeling for complex environments.
- Future research directions and emerging trends in GRL.
How This Book Was Generated
This book is the result of our advanced AI text generator, meticulously crafted to deliver not just information but meaningful insights. By leveraging our AI book generator, cutting-edge models, and real-time research, we ensure each page reflects the most current and reliable knowledge. Our AI processes vast data with unmatched precision, producing over 200 pages of coherent, authoritative content. This isn’t just a collection of facts—it’s a thoughtfully crafted narrative, shaped by our technology, that engages the mind and resonates with the reader, offering a deep, trustworthy exploration of the subject.
Satisfaction Guaranteed: Try It Risk-Free
We invite you to try it out for yourself, backed by our no-questions-asked money-back guarantee. If you're not completely satisfied, we'll refund your purchase—no strings attached.